กรณีศึกษา Gmail ยอมขาดทุน เพื่อใหญ่กว่าเดิม /โดย ลงทุนแมน
ในหนึ่งวัน มนุษย์รับส่งอีเมลกัน 293,000 ล้านฉบับ
หรือเฉลี่ย 38 ฉบับต่อคน
ธุรกิจให้บริการแพลตฟอร์มอีเมล จึงมีความสำคัญต่อโลกในยุคนี้เป็นอย่างมาก
ถ้าถามว่า คนใช้อีเมลอะไรมากที่สุด
เมื่อ 20 ปีที่แล้ว คงจะเป็น Hotmail ของ Microsoft
แต่ในปัจจุบัน คนส่วนใหญ่หันมาใช้ “Gmail” ของ Google กันแทน
เพราะอะไร Gmail ถึงแย่งส่วนแบ่งตลาดมาจาก Hotmail ได้สำเร็จ
ลงทุนแมนจะเล่าให้ฟัง
┏━━━━━━━━━━━━┓
Blockdit โซเชียลมีเดีย รูปแบบใหม่
Blockdit.com/download
┗━━━━━━━━━━━━┛
Google เริ่มศึกษาธุรกิจอีเมลมาตั้งแต่ปี 1999
ซึ่งขณะนั้น ผู้นำตลาดคือ Hotmail ของ Microsoft มีสมาชิกอยู่ราว 30 ล้านบัญชี
ต่อมาในวันที่ 1 เมษายน 2004 Google ก็ได้เปิดตัวบริการอีเมลฟรี ชื่อว่า “Gmail”
ตอนแรกผู้คนต่างคิดว่าเป็นเรื่องโกหกฉลองวัน April Fool’s Day
เพราะสิ่งที่ Google นำเสนอ มันดูน่าเหลือเชื่อมากสำหรับยุคนั้น
โดย Gmail ให้พื้นที่เก็บข้อมูลถึง 1,000 MB หรือ 1 GB
สูงกว่าผู้เล่นรายอื่นที่ให้แค่ประมาณ 4 MB
ทำให้สมาชิกไม่ต้องคอยลบอีเมลอยู่บ่อยครั้ง
ถือว่าแก้ปัญหา pain point ของคนที่ใช้อีเมลในยุคนั้นได้อย่างดี
นอกจากนี้ Gmail ยังได้มุ่งเน้นพัฒนาระบบต่างๆ อย่างต่อเนื่อง เพื่อสร้างประสบการณ์ที่ดีในการใช้อีเมล
ไม่ว่าจะเป็น การใช้ฟีเจอร์คล้ายเว็บไซต์ Google ค้นหาข้อมูลภายในอีเมลได้ละเอียด
จากเดิมที่เราค้นหาอีเมลยาก
มาตอนนี้เราสามารถหาอีเมลด้วยระบบ algorithm ที่ฉลาดของ Google
และการพัฒนาต่อมาก็คือ การเชื่อมต่อเข้ากับแพลตฟอร์มอื่นๆ เช่น Google Drive ช่วยให้รับส่งไฟล์ และทำงานสะดวกขึ้น
จนในปัจจุบัน Google ได้เริ่มใช้ Machine Learning ของ AI มาคาดเดาประโยคที่เรากำลังจะพิมพ์เลยทีเดียว
ด้วยคุณภาพของแพลตฟอร์ม ที่ส่งเสริมประสิทธิภาพการใช้อีเมล
จึงทำให้ Gmail ได้รับความนิยม จนมีจำนวนสมาชิกแซงหน้า Hotmail และครองส่วนแบ่งตลาดในที่สุด
ปี 2011
Gmail มีสมาชิก 220 ล้านบัญชี
Hotmail มีสมาชิก 360 ล้านบัญชี
ปี 2019
Gmail มีสมาชิก 1,500 ล้านบัญชี
Outlook (แพลตฟอร์มใหม่แทนที่ Hotmail) มีสมาชิก 400 ล้านบัญชี
อ่านถึงตรงนี้ หลายคนอาจจะสงสัย
ว่าทำไม Microsoft ถึงไม่พัฒนาฟีเจอร์ของ Hotmail ให้เป็นเช่นนั้น ทั้งที่บริษัทก็มีทรัพยากรมากมายที่จะมาแข่งขันกับ Google
คำตอบคือ 2 บริษัทนี้ ใช้กลยุทธ์ในการบริหารธุรกิจอีเมลแตกต่างกัน
ธุรกิจ Hotmail สามารถสร้างรายได้ให้ตัวเอง
โดยเงินที่ใช้พัฒนาธุรกิจ ก็จะมาจากค่าโฆษณาบนหน้าแพลตฟอร์มของ Hotmail
แต่ Google สร้าง Gmail ให้เป็นส่วนหนึ่งของภาพใหญ่
ไม่ได้ให้ Gmail หารายได้โดยตรง
Google เก็บข้อมูลผู้ใช้อีเมล แล้วนำไปวิเคราะห์เพื่อการโฆษณาบนที่อื่น เช่น Search Engine ของตนเอง
เพราะพวกเขามองว่า คนจะกดดูโฆษณาตอนค้นหาข้อมูล มากกว่าตอนเช็กอีเมล
ดังนั้น Gmail จะสร้างมูลค่าเพิ่มต่อแพลตฟอร์มหลักของบริษัท มากกว่าตัวมันเอง
ทำให้ Google พัฒนา Gmail ได้เต็มที่ ทั้งให้เนื้อที่จำนวนมาก และไม่มีโฆษณา โดยไม่ถูกจำกัดว่าผลิตภัณฑ์นี้จะขาดทุนหรือไม่ เพราะบริษัทมีรายได้จากค่าโฆษณาใน Search Engine มหาศาลอยู่แล้ว
และถึงแม้ต่อมา คู่แข่งรายอื่นจะเสนอฟีเจอร์ต่างๆ ขึ้นมาทัดเทียมกัน ก็ไม่อาจดึงดูดคนไปจาก Gmail ได้ เพราะคนคุ้นชิน และไม่มีเหตุผลจูงใจมากพอในการเปลี่ยนไปอีกแพลตฟอร์มหนึ่ง
ในทางทฤษฎี เราสามารถสมัครอีเมลใหม่ได้ทุกเมื่อ
แต่ในทางปฏิบัติ เราคงไม่ได้เปลี่ยนอีเมลกันบ่อยๆ เพราะเราได้ใช้มันเป็นบัญชีในการติดต่อสื่อสารกับผู้อื่นมากมายไปแล้ว ทำให้ธุรกิจนี้มี Barriers to Entry อยู่พอสมควร
ในขณะที่คู่แข่งรายอื่นก็ไม่สามารถยอมขาดทุนได้แบบ Google เพราะเขาเหล่านั้นไม่มีแพลตฟอร์มหลักในการหารายได้
อย่างไรก็ตาม การเก็บข้อมูลส่วนตัวจากอีเมล ส่งผลให้เกิดประเด็นถกเถียงด้านความเหมาะสม
จนเมื่อปี 2017 นั้น Google ก็ได้ประกาศยุติใช้ข้อมูลจาก Gmail มาวิเคราะห์เพื่อการโฆษณา
ในตอนนี้ Google จึงเน้นให้ Gmail เป็นหน้า portal นำไปสู่การใช้ผลิตภัณฑ์อื่นของ Google เช่น การซื้อเนื้อที่เพิ่มจาก Google Drive หรือ การใช้ Docs, Sheets, Slides และในช่วงหลังรายได้จากผลิตภัณฑ์เหล่านี้ที่เรียกว่า Cloud ก็เติบโตได้ดีเช่นกัน
จากเรื่องนี้ ทำให้เห็นว่า
ในบางครั้ง มูลค่าเชิงธุรกิจของผลิตภัณฑ์
ไม่ได้อยู่ที่ว่า ตัวมันเอง สร้างผลตอบแทนเป็นตัวเงินเท่าไหร่
แต่มูลค่าที่แท้จริงอาจซ่อนอยู่ ซึ่งสร้างประโยชน์ในภาพรวมให้เราได้มากกว่า
แนวคิดนี้ Google ยังนำไปใช้กับผลิตภัณฑ์อื่น ไม่ใช่เฉพาะ Gmail
ตัวอย่างที่เราเห็นได้ชัดก็คือ Google Maps
เคยสงสัยไหม ว่าเราใช้แผนที่ของ Google ฟรี แล้ว Google ได้อะไร
คำตอบคือ Google ไม่มีรายได้โดยตรง
แต่มูลค่ามหาศาลที่ซ่อนอยู่ก็คือ
ข้อมูลว่าเราไปไหนบ้างในแต่ละวัน
ถ้าวันนี้มีบางอย่างที่ตัวเรารู้สึกว่า เราทำไปแล้วไม่ได้อะไร
ลองคิดดูดีๆ
สิ่งนั้นมันอาจจะมีประโยชน์ต่อเราในภาพรวมก็เป็นได้
ยอมขาดทุนสิ่งหนึ่ง เพื่อสิ่งใหม่ที่ใหญ่กว่าเดิม..
┏━━━━━━━━━━━━┓
Blockdit โซเชียลมีเดีย รูปแบบใหม่
Blockdit.com/download
┗━━━━━━━━━━━━┛
References
-https://www.express.co.uk/…/Gmail-account-Google-app-Micros…
-https://www.cnbc.com/…/how-gmail-and-google-beat-yahoo-and-…
-https://en.wikipedia.org/wiki/Gmail
-https://finance.yahoo.com/quote/GOOG/
-https://www.lifewire.com/how-many-emails-are-sent-every-day…
-https://variety.com/…/n…/google-gmail-ads-emails-1202477321/
同時也有4部Youtube影片,追蹤數超過40萬的網紅我要做富翁,也在其Youtube影片中提到,大家都知道 AI 人工智能是未來發展的大方向,未來的電腦除了可以自動駕駛汽車、醫療、法律之外,在投資領域又是否能幫一般人賺大錢?今集 #我要做程式交易,Eva 會簡單講解一下 AI、Machine Learning、Deep Learnin、Big Data 等,以及分析一下,這些「未來」科技,如何...
「machine learning algorithm」的推薦目錄:
- 關於machine learning algorithm 在 ลงทุนแมน Facebook
- 關於machine learning algorithm 在 Huyen Chip Facebook
- 關於machine learning algorithm 在 โปรแกรมเมอร์ไทย Thai programmer Facebook
- 關於machine learning algorithm 在 我要做富翁 Youtube
- 關於machine learning algorithm 在 DroidSans Youtube
- 關於machine learning algorithm 在 珊蒂微AI Youtube
machine learning algorithm 在 Huyen Chip Facebook 八卦
Làm thế nào để học miễn phí các khoá học ở Stanford
Mình muốn chia sẻ với các bạn một điều mà không phải ai cũng biết, đó là nội dung hầu hết các khoá học ở Stanford được đưa lên mạng miễn phí. Các lớp học thường có website để sinh viên tiện tìm thông tin, và website đó thường có đầy đủ thông tin về bài giảng, bài tập về nhà, sách giáo khoa., ai cũng có thể vào xem.
Website các lớp học ở stanford thường có url dạng web.stanford.edu/class/[mã lớp học]. Ví dụ, lớp của mình có mã CS20, website của nó là web.stanford.edu/class/cs20. Lớp về machine learning nổi tiếng của Andrew Ng có mã là CS229, và website là web.stanford.edu/class/cs229
Để biết mã các lớp học, bạn có thể vào explorecourses.stanford.edu Đây là trang danh mục các lớp học ở Stanford. Bạn có thể điền từ khoá để tìm. Ví dụ, bạn muốn học các lớp data science thì điền từ khoá data science, nó sẽ hiện ra thông tin các lớp học về data science như mã lớp học, tên lớp học, người dạy, nội dung giới thiệu lớp học. Bạn cũng có thể tìm các lớp học theo nghành.
Đây là danh sách mã một số lớp học ở Stanford mà mình rất thích.
Lập trình cơ bản: CS106A, CS106B
Hệ thống: CS107, CS110
Probability: CS109
Algorithm: CS161, CS168 (lớp của thầy siêu dễ thương)
An ninh mạng: CS255
Network: CS155
Decision making under uncertainty (đưa ra quyết định khi không đủ thông tin): CS238 (mình quý ông thầy lớp này kinh khủng)
Cryptocurrency (bitcoin các thứ): CS251
Xử lý dữ liệu khổng lồ: CS246
Khoá dạy về machine learning cho computer vision thần thánh: CS231N
Khoá dạy về machine learning cho natural language processing: CS224N
Khoá phân tích mạng lưới (analysis of network): CS224W
Các ngành không phải khoa học máy tính thì thường ít có website cho người ngoài (họ sử dụng website nội bộ). Nhưng nếu bạn tìm được lớp nào hay ho qua trang explorecourses, hãy thử tìm qua mạng xem có website của lớp đó khônh nhé.
Chúc mọi người tự học hiệu quả!
machine learning algorithm 在 โปรแกรมเมอร์ไทย Thai programmer Facebook 八卦
ในวิชา "วิทยาการคำนวณ" ระดับชั้น ม. 5
ได้ดึงวิชา data science (วิทยาศาสตร์ข้อมูล)
มาปูพื้นฐานให้เด็กๆ ได้เรียนกันแล้ว นับว่าเป็นโชคดี
เพราะวิชาพวกนี้เป็นของสูง กว่าจะสัมผัสก็คงตอนป.ตรี โท เอก
...Continue ReadingIn the subject of ′′ Calculation Theology ′′ class. 5
Pulled data science (data science)
Let's master the foundation for kids to learn. It's considered lucky.
Because these subjects are high to touch. It's probably in the middle of the year. Tri To Aek
Which I will review the content to read roughly. The content is divided into 4 chapters.
.
👉 ++++ Chapter 1-Information is valuable +++++
.
Data science in the textbook. Used by Thai name as ′′ Information Science ′′
This chapter will mention Big Data or big data with lots of valuable information.
And so much role in this 4.0 s both public and private sector.
.
If you can't imagine when you played Google search network, you'll find a lot of information that you can use in our business. This is why data science plays a very important role.
.
It's not surprising that it makes the Data Scientist s' career (British name data scientist) play the most important role and charming and interesting profession of the 21th century.
.
Data science, if in the book, he defines it
′′ Study of the process, method or technique to process enormous amounts of data to process to obtain knowledge, understand phenomena, or interpret prediction or prediction, find out patterns or trends from information.
and can be analysed to advise the right choice or take decision for maximum benefit
.
For Data science work, he will have the following steps.
- Questioning my own interest.
- Collect information.
- Data Survey
- Data Analysis (analyze the data)
- Communication and Results Visualization (Communicate and visualize the results)
.
🤔 Also he talks about design thinking... but what is it?
Must say the job of a data scientist
It doesn't end just taking the data we analyzed.
Let's show people how to understand.
.
The application design process is still required.
To use data from our analytics
The word design thinking is the idea. The more good designer it is.
Which Data Scientists Should Have To Design Final Applications
Will meet user demand
.
👉 ++++ Chapter 2 Collection and Exploration +++++
.
This chapter is just going to base.
2.1 Collection of data
In this chapter, I will talk about information that is a virtual thing.
We need to use this internet.
2.2 Data preparation (data preparation)
Content will be available.
- Data Cleaning (data cleansing)
- Data Transformation (data transformation)
In the university. 5 is not much but if in college level, you will find advanced technique like PCA.
- Info Link (combining data)
2.3 Data Exploration (data exploration)
Speaking of using graphs, let's explore the information e
Histogram graph. Box plot diagram (box plot). Distributed diagram (scatter plot)
With an example of programming, pulls out the plot to graph from csv (or xls) file.
2.4 Personal Information
For this topic, if a data scientist is implementing personal data, it must be kept secret.
.
Where the issues of personal information are now available. Personal Data Protection is Done
.
.
👉 ++++ Chapter 3 Data Analysis ++++
.
Divided into 2 parts:
.
3.1 descriptive analysis (descriptive analytics)
Analyzing using the numbers we've studied since
- Proportion or percentage
- Medium measurement of data, average, popular base.
Correlation (Correlation) relationship with programming is easy.
.
.
3.2 predictive analysis (predictive analytics)
.
- numeric prediction is discussed. (numeric prediction)
- Speaking of technique linear regression, a straight line equation that will predict future information.
Including sum of squared errors
Let's see if the straight line graph is fit with the information. (with programming samples)
- Finally mentioned K-NN (K-Nearest Neighbors: K-NN) is the closest way to finding K-N-Neighborhood for classification (Category)
*** Note *****
linear regression กับ K-NN
This is also an algorithm. One of the machine learning (machine learning, one branch of AI)
Kids in the middle of the day, I get to study.
.
.
👉 +++ Chapter 4 Making information pictured and communicating with information +++
.
This chapter doesn't matter much. Think about the scientist after analyzing what data is done. The end is showing it to other people by doing data visualization. (Better summoning)
.
In contents, it's for example using a stick chart, line chart, circular chart, distribution plan.
.
The last thing I can't do is tell a story from information (data story telling) with a message. Be careful when you present information.
.
.
.
*** this note ***
😗 Program language which textbooks mentioned and for example.
It's also python and R language
.
For R language, many people may not be familiar.
The IT graduate may be more familiar with Python.
But anyone from the record line will surely be familiar.
Because R language is very popular in statistical line
And it can be used in data science. Easy and popular. Python
.
But if people from data science move to another line of AI
It's deep learning (deep learning)
Python will be popular with eating.
.
.
#########
😓 Ending. Even I wrote a review myself, I still feel that.
- The university. 5 is it going to be hard? Can a child imagine? What did she do?
- Or was it right that I packed this course into Big Data era?
You can comment.
.
But for sure, both parents and teachers are tired.
Because it's a new content. It's real.
Keep fighting. Thai kids 4.0
.
Note in the review section of the university's textbook. 4 There will be 3 chapters. Read at.
https://www.facebook.com/programmerthai/photos/a.1406027003020480/2403432436613260/?type=3&theater
.
++++++++++++++++++++
Before leaving, let's ask for publicity.
++++++++++++++++++++
Recommend the book ′′ Artificial Intelligence (AI) is not difficult ′′
It can be understood by the number. End of book 1 (Thai language content)
Best seller ranked
In the MEB computer book category.
.
The contents will describe Artificial Intelligence (A) in view of the number. The end.
Without a code of dizzy
With colorful illustrations to see, easy to read.
.
If you are interested, you can order.
👉 https://www.mebmarket.com/web/index.php?action=BookDetails&data=YToyOntzOjc6InVzZXJfaWQiO3M6NzoiMTcyNTQ4MyI7czo3OiJib29rX2lkIjtzOjY6IjEwODI0NiI7fQ&fbclid=IwAR11zxJea0OnJy5tbfIlSxo4UQmsemh_8TuBF0ddjJQzzliMFFoFz1AtTo4
.
Personal like the book. You can see this link.
👉 https://www.dropbox.com/s/fg8l38hc0k9b0md/chapter_example.pdf?dl=0
.
Sorry, paper book. I don't have it yet. Sorry.
.
✍ Written by Thai programmer thai progammerTranslated
machine learning algorithm 在 我要做富翁 Youtube 的評價
大家都知道 AI 人工智能是未來發展的大方向,未來的電腦除了可以自動駕駛汽車、醫療、法律之外,在投資領域又是否能幫一般人賺大錢?今集 #我要做程式交易,Eva 會簡單講解一下 AI、Machine Learning、Deep Learnin、Big Data 等,以及分析一下,這些「未來」科技,如何影響一般人的投資策略。
======================
1) 程式交易新手會+試堂:https://edu.money-tab.com/eva-algo-sharing
2) 我要做股神APP下載:http://onelink.to/mtapp
3) 緊貼我們社交平台,不錯過任何免費分析/教學:
YouTube頻道: https://youtube.com/channel/UCdWNwPuaS1o2dIzugNMXWtw?sub_confirmation=1
Facebook專頁:https://facebook.com/203349819681082
#大數據 #深度學習 #人工智能 #machine learning #量化分析
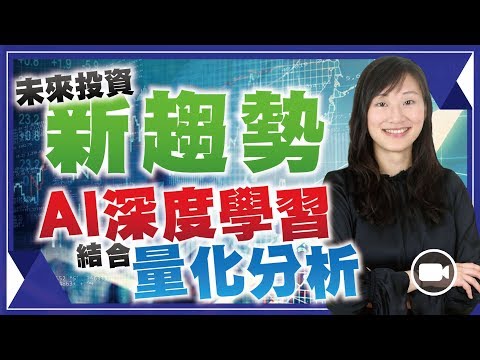
machine learning algorithm 在 DroidSans Youtube 的評價
เหล่านักวิจัยจาก Google แผนกเทคโนโลยีด้านสุขภาพ ค้นพบวิธีใหม่ในการตรวจหาความเสี่ยงในการเกิดโรคหัวใจของผู้ป่วย โดยการใช้ระบบ AI แบบ machine learning เพื่อสแกนบริเวณด้านหลังของดวงตาและทำการวิเคราะห์ถึงความเสี่ยงในการเกิดโรคหัวใจของผู้ป่วยคนนั้นได้ ซึ่งวิธีดังกล่าวมีความแม่นยำพอๆ กับวิธีปกติที่แพทย์ทั่วไปใช้เลยนะ
รายละเอียดเพิ่มเติม https://droidsans.com/google-verily-ai-algorithm-eye-scan-heart-disease-risk/
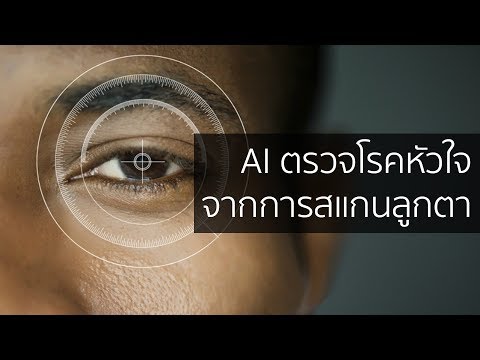
machine learning algorithm 在 珊蒂微AI Youtube 的評價
如果連我們人類都無法掌握那個可以創造「完美笑點」的神奇公式,那我們要怎麼讓教好人工智慧的「幽默感」、怎麼教人工智慧「講笑話」呢?
從博恩講笑話的事件|簡單聊聊|人工智慧懂不懂幽默感?|【珊蒂微AI】
【參考資料】
🔗美國研究員Janelle Shane嘗試使用43,000 個笑話來訓練神經網絡學會幽默:“Machine learning failures - for art!” by Janelle Shane: https://www.youtube.com/watch?v=yneJIxOdMX4
🔗Janelle Shane的部落格:lewisandquark.tumblr.com
🔗牛津大學,微軟研究院和TRASH 的一組研究人員開展了一項調查詞彙嵌入中幽默的研究。:論文地址:https://arxiv.org/pdf/1902.02783.pdf
🔗What Can We Learn From Computers (NOT) Understanding Humor - Julia Taylor Rayz:https://www.youtube.com/watch?v=Vy8WiKvT4gY
🔗Can artificial intelligence be taught how to joke?: https://heartbeat.fritz.ai/can-artificial-intelligence-be-taught-how-to-joke-7c7d53a3492a
🔗A robot walks into a bar, doesn’t get the joke:https://www.youtube.com/watch?v=7z7Dl61rgA0
🔗The Science of Humor Is No Laughing Matter: https://www.psychologicalscience.org/observer/the-science-of-humor-is-no-laughing-matter
🔗Machines need an algorithm for humor. This is what it looks like | Vinith Misra | TED Institute:https://www.youtube.com/watch?v=2X3TF_J31is
🔗利用AI在線上寫唐詩宋詞:
https://www.popmars.com/ai/%E4%BA%BA%E5%B7%A5%E6%99%BA%E8%83%BD%E4%BD%A0%E4%B9%9F%E5%8F%AF%E4%BB%A5%E6%98%AF%E5%A4%A7%E6%96%87%E8%B1%AA%EF%BC%8C%E5%88%A9%E7%94%A8%E4%BA%BA%E5%B7%A5%E6%99%BA%E8%83%BD%E5%9C%A8%E7%BA%BF%E5%86%99/
#博恩鄭南榕
#人工智慧與幽默感
#珊蒂微AI

machine learning algorithm 在 Machine learning - Wikipedia 的相關結果
It is seen as a part of artificial intelligence. Machine learning algorithms build a model based on sample data, known as training data ... ... <看更多>
machine learning algorithm 在 A Tour of Machine Learning Algorithms 的相關結果
A model is prepared through a training process in which it is required to make predictions and is corrected when those predictions are wrong. ... <看更多>
machine learning algorithm 在 Commonly Used Machine Learning Algorithms | Data Science 的相關結果
Commonly used Machine Learning Algorithms (with Python and R Codes) · 1. Linear Regression · 2. Logistic Regression · 3. Decision Tree · 4. SVM ( ... ... <看更多>